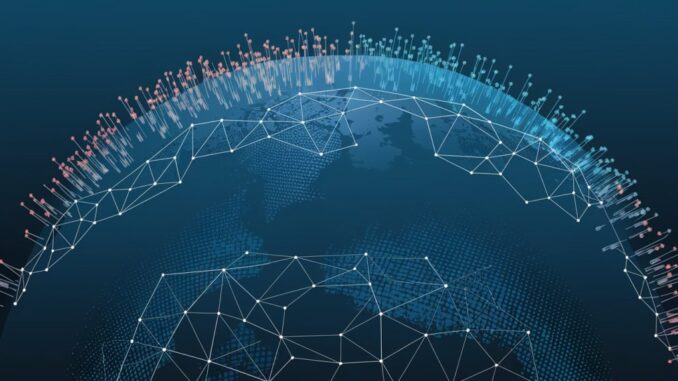
Subscribe to our daily and weekly newsletters for the most recent updates and exclusive material on leading AI coverage. Discover More
Recent research from Shanghai Jiao Tong University indicates that large language models (LLMs) are capable of mastering intricate reasoning tasks without depending on extensive datasets. The study reveals that even with a limited selection of well-selected examples, one can train an LLM for activities previously believed to necessitate tens of thousands of training instances.
This effectiveness stems from the fundamental knowledge that contemporary LLMs acquire during the pre-training phase. As new training techniques become increasingly efficient in terms of data and computation, businesses might have the capability to develop tailored models without needing access to the extensive resources of large artificial intelligence laboratories.
Less is more (LIMO)
The researchers, in their investigation, question the notion that a substantial amount of data is essential to train LLMs for reasoning activities. They propose the idea of “less is more” (LIMO). Their research builds on earlier findings that established LLMs could align with human preferences using merely a few examples.
Their experiments illustrated that a LIMO dataset for intricate mathematical reasoning tasks could be constructed with just a few hundred training instances. An LLM that was fine-tuned on this dataset was able to produce sophisticated chain-of-thought (CoT) reasoning sequences that allowed it to achieve tasks with an impressive success rate.
For instance, a Qwen2.5-32B-Instruct model fine-tuned using 817 training instances selected based on LIMO attained an accuracy of 57.1% on the highly demanding AIME benchmark and 94.8% on MATH, surpassing models trained on a hundred times more examples. It also outperformed reasoning models like QwQ-32B-Preview (a version of the Qwen model tailored for reasoning) and OpenAI o1-preview, both of which were trained using larger datasets and computational resources.
Additionally, LIMO-trained models are capable of generalizing to examples that are significantly different from their training data. For example, on the OlympiadBench scientific benchmark, the LIMO model exceeded QwQ-32B-Preview’s performance, and on the challenging GPQA benchmark, it achieved 66.7% accuracy, close to OpenAI-o1-preview’s highest score of 73.3%.
What does it signify for enterprise AI?
Tailoring LLMs presents an appealing use case for enterprise scenarios. With techniques like retrieval-augmented generation (RAG) and in-context learning, LLMs can be customized to utilize unique data or tackle new tasks without incurring the costs of extensive fine-tuning.
Nevertheless, reasoning activities frequently necessitate the training and fine-tuning of LLMs. The prevailing belief has been that such tasks require large quantities of training examples featuring highly detailed reasoning chains and solutions. Developing such datasets is time-consuming and impractical for many applications and organizations.
In recent times, researchers have demonstrated that pure reinforcement learning methodologies can empower models to self-train for reasoning tasks by generating numerous solutions and selecting the optimal ones. While this method requires less manual intervention, it still demands costly computational resources that many enterprises find inaccessible.
Conversely, producing a few hundred examples is a task that many companies can manage, making specialized reasoning models more attainable for a broader array of organizations.
“This revelation has significant implications for artificial intelligence research: It suggests that even high-competition complex reasoning skills can be effectively drawn out using minimal but carefully selected training samples,” the researchers state.
Reasons LIMO functions
The researchers identified two major factors in their experiments explaining why LLMs can grasp complex reasoning tasks with fewer examples.
Firstly, cutting-edge foundation models have been exposed to a vast quantity of mathematical content and code during the pre-training stage. This indicates that these LLMs already possess rich reasoning knowledge embedded in their parameters that can be activated through thoughtfully designed examples.
Secondly, recent post-training methodologies have shown that permitting models to generate extended reasoning sequences greatly enhances their reasoning capacity. Essentially, allowing models more time to “think” enables them to unpack and utilize their pre-trained knowledge more efficiently.
“We propose that successful reasoning derives from the combination of these two factors: extensive pre-trained knowledge and adequate computational resources during inference,” the researchers comment. “These advancements collectively indicate a striking possibility: If models hold extensive reasoning knowledge and are provided sufficient computational scope, then activating their reasoning faculties may necessitate merely a handful of high-quality training samples that foster extended deliberation, instead of extensive fine-tuning datasets.”

The findings of the researchers indicate that creating effective LIMO datasets depends on identifying the appropriate problems and solutions. Data curators should focus on selecting challenging problems that necessitate complex reasoning chains, diverse thought processes, and knowledge integration. The problems chosen should also diverge from the model’s training distribution to encourage the exploration of new reasoning strategies and push it towards generalization.
Consequently, solutions should be clearly structured and well-organized, with reasoning steps tailored to the complexity of the problem. High-quality solutions ought to provide strategic educational assistance by progressively enhancing understanding through carefully formed explanations.
“By concentrating on a minimal yet scrupulously curated set of reasoning chains, we embody the core tenet of LIMO: Quality demonstrations, rather than sheer data quantity, are essential for unlocking complex reasoning capabilities,” the researchers assert.
The researchers have made available the code and data utilized in training the LIMO models during their experiments. In the future, they aim to broaden the concept to additional domains and applications.
Be the first to comment