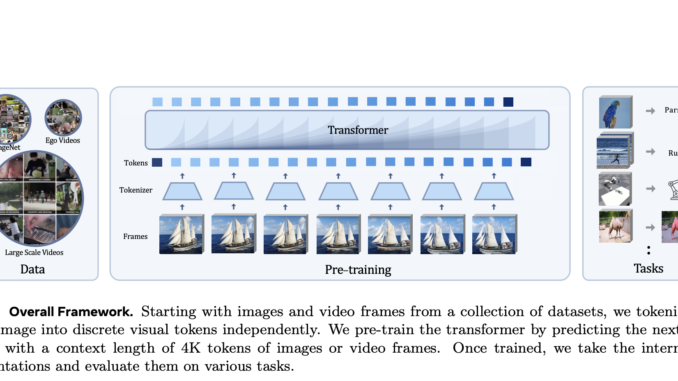
The Revolutionary AI Model
Autoregressive pre-training has been demonstrated to be transformative in the realm of machine learning, particularly in relation to sequential data handling. Predictive modeling of subsequent sequence elements has proven to be highly effective in natural language processing and, increasingly, has been investigated within the field of computer vision. Video modeling stands out as a sector that remains largely untapped, presenting opportunities for advancements in action recognition, object tracking, and robotics applications. These innovations are attributed to expanding datasets and advancements in transformer architectures that regard visual inputs as organized tokens apt for autoregressive training.
Modeling videos introduces distinct hurdles due to their temporal dynamics and redundancy. Unlike text, which follows a definite sequence, video frames often encompass redundant information, complicating the tokenization process and the development of suitable representations. Effective video modeling should successfully navigate this redundancy while capturing spatiotemporal relationships across frames. Most frameworks have concentrated on image-based representations, leaving the enhancement of video architectures unaddressed. This challenge necessitates new techniques to harmonize efficiency and performance, especially when considering video forecasting and robotic manipulation.
Visual representation learning through convolutional networks and masked autoencoders has shown success in image-related tasks. However, these strategies often fall short in video applications as they struggle to fully convey temporal dependencies. Tokenization techniques such as dVAE and VQGAN generally transform visual information into tokens, and while they have demonstrated some efficacy, scaling this approach becomes problematic in contexts with mixed datasets that include both images and videos. Patch-based tokenization does not sufficiently generalize to handle various tasks in an effective manner within a video context.
A research group from Meta FAIR and UC Berkeley has launched the Toto series of autoregressive video models. Their innovation lies in addressing the limitations of conventional methods, treating videos as sequences of discrete visual tokens and utilizing causal transformer architectures to anticipate subsequent tokens. The researchers constructed models capable of seamlessly integrating image and video training by utilizing a unified dataset that contains over one trillion tokens from both images and videos. This cohesive strategy enabled the team to leverage the advantages of autoregressive pre-training across both domains.
The Toto models employ dVAE tokenization with an 8k-token vocabulary to analyze images and video frames. Each frame is resized and tokenized independently, resulting in sequences comprised of 256 tokens. These tokens are subsequently processed by a causal transformer that incorporates the features of RMSNorm and RoPE embeddings to achieve enhanced AI model performance. The training was conducted on ImageNet and HowTo100M datasets, with tokenization occurring at a resolution of 128×128 pixels. Additionally, the researchers fine-tuned the models for downstream objectives by substituting average pooling with attention pooling to enhance the quality of representation.
The models exhibit strong performance across the benchmarks. In ImageNet classification, the largest Toto model achieved a top-1 accuracy of 75.3%, surpassing other generative models such as MAE and iGPT. In the Kinetics-400 action recognition task, the models attained a top-1 accuracy of 74.4%, showcasing their ability to comprehend intricate temporal dynamics. On the DAVIS dataset for semi-supervised video tracking, the models achieved J&F scores of up to 62.4, thereby improving upon previous state-of-the-art benchmarks set by DINO and MAE. Furthermore, in robotics tasks such as object manipulation, Toto models exhibit faster learning rates and greater sample efficiency. For instance, the Toto-base model successfully executed a cube-picking real-world task on the Franka robot with an accuracy rate of 63%. Overall, these results are remarkable in terms of the adaptability and scalability of these proposed models across varied applications.
This research has made notable advancements in video modeling by tackling redundancy and tokenization challenges. The researchers effectively demonstrated that “through unified training on both images and videos, this type of autoregressive pretraining is typically efficient across various tasks.” Innovative architecture and tokenization methodologies lay the groundwork for further research in dense prediction and recognition. This represents a significant stride toward realizing the full potential of video modeling for practical applications.
Explore the Paper and Project Page. All acknowledgment for this research is attributable to the researchers involved in this project. Additionally, do not forget to follow us on Twitter and join our Telegram Channel and LinkedIn Group. Remember to join our 65k+ ML SubReddit.
🚨 FREE UPCOMING AI WEBINAR (JAN 15, 2025): Enhance LLM Accuracy with Synthetic Data and Evaluation Intelligence–Participate in this webinar to acquire actionable insights on enhancing LLM model performance and accuracy while safeguarding data privacy.
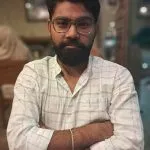
Nikhil serves as an intern consultant at Marktechpost. He is currently engaged in a joint dual degree in Materials at the Indian Institute of Technology, Kharagpur. Nikhil has a passion for AI/ML and consistently researches usage in areas such as biomaterials and biomedical sciences. Possessing a robust foundation in Material Science, he is investigating novel advancements and creating chances to make contributions.
Be the first to comment