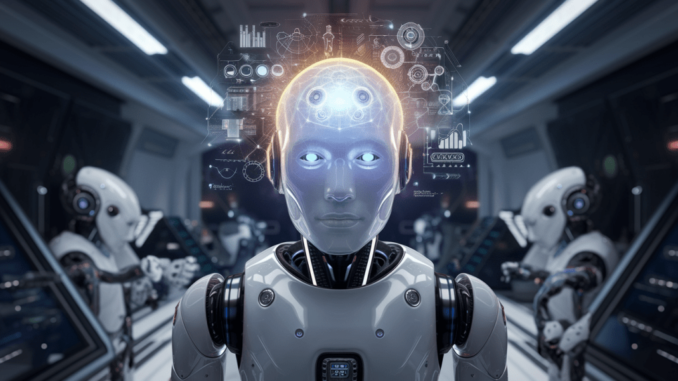
Cache Augmented technology is transforming the manner in which we conceive data storage and retrieval.
Overview of Cache-Augmented Generation (CAG)
Grasping the methodology of cache generation, along with Cache Augmented strategies, is essential for web developers and IT specialists to guarantee effective data retrieval and optimal system performance.
Subscribe to our daily and weekly newsletters for the most recent updates and exclusive material on industry-leading AI insights. Discover More
Retrieval-augmented generation (RAG) has become the standard method for tailoring large language models (LLMs) to retrieve specific information. However, RAG incurs initial technical expenses and can be sluggish. Now, due to advancements in long-context LLMs, businesses can circumvent RAG by embedding all proprietary information directly into the prompt.
A recent investigation by the National Chengchi University in Taiwan illustrates that by utilizing long-context LLMs along with caching methods, one can develop customized applications that outperform RAG frameworks. Referred to as cache-augmented generation (CAG), this strategy could serve as a straightforward and effective substitute for RAG in enterprise environments where the knowledge base can fit within the model’s context window.
Drawbacks of RAG
RAG is a potent approach for addressing open-domain inquiries and specialized assignments. It employs retrieval algorithms to collect documents pertinent to the inquiry and adds context to assist the LLM in formulating more precise responses.
Nevertheless, RAG introduces multiple drawbacks to LLM applications. The additional retrieval phase injects latency that may diminish the user experience. The outcome also relies on the caliber of the document selection and ranking process. In numerous instances, the limitations of the models utilized for retrieval necessitate that documents be divided into smaller segments, which can negatively impact the retrieval process.
Furthermore, RAG generally complicates the LLM application, necessitating the development, integration, and upkeep of supplementary components. This extra burden can decelerate the development cycle.
Cache-Augmented Retrieval
The alternative to constructing a RAG pipeline is to incorporate the complete document collection into the prompt, allowing the model to identify which segments are pertinent to the request. This technique alleviates the complications associated with the RAG pipeline and the issues arising from retrieval inaccuracies.
However, there are three principal challenges when front-loading all documents into the prompt. Firstly, lengthy prompts will hinder the model’s speed and elevate inference costs. Secondly, the dimensions of the LLM’s context window impose constraints on the number of documents that can be included in the prompt. Lastly, introducing irrelevant data into the prompt can perplex the model and diminish the quality of its responses. Consequently, simply cramming all your documents into the prompt, instead of selecting the most pertinent ones, may ultimately degrade the model’s efficacy.
How CAG Overcomes Challenges
The proposed CAG methodology harnesses three significant trends to navigate these challenges.
Initially, sophisticated caching techniques are rendering the processing of prompt templates faster and more economical. The fundamental concept of CAG is that the knowledge documents are integrated into every prompt directed to the model. Thus, attention values of their tokens can be calculated ahead of time, rather than upon receiving requests. This pre-computation curtails the duration required to process user inquiries.
Leading LLM providers such as OpenAI, Anthropic, and Google offer prompt caching capabilities for the repetitive elements of your prompt, which can encompass the knowledge documents and directives included at the start of your prompt. With Anthropic, you can diminish costs by as much as 90% and reduce latency by 85% for the cached elements of your prompt. Similar caching functionalities have been developed for open-source LLM-hosting platforms.
Secondly, long-context LLMs are facilitating the inclusion of more documents and knowledge within prompts. Claude 3.5 Sonnet accommodates up to 200,000 tokens, while GPT-4o allows for 128,000 tokens, and Gemini up to 2 million tokens. This capacity enables the integration of multiple documents or entire texts into the prompt.
Lastly, advanced training methodologies are empowering models to improve retrieval, reasoning, and question-answering on significantly lengthy sequences. Over the past year, researchers have introduced various LLM benchmarks for long-sequence challenges, including BABILong, LongICLBench, and RULER. These benchmarks assess LLMs on complex tasks such as multiple retrieval and multi-hop question-answering. While there remains room for enhancement in this domain, AI laboratories persist in making strides.
As newer iterations of models continue to extend their context windows, they will be capable of processing more extensive knowledge collections. Furthermore, we can anticipate ongoing improvements in models’ abilities to extract and utilize pertinent information from lengthy contexts.
“These two trends will substantially enhance the practicality of our approach, allowing it to accommodate more intricate and diverse applications,” the researchers assert. “As a result, our methodology is well-situated to emerge as a robust and versatile solution for knowledge-intensive tasks, capitalizing on the expanding capacities of next-generation LLMs.”
RAG vs CAG
To evaluate RAG and CAG, the researchers conducted experiments using two renowned question-answering benchmarks: SQuAD, which concentrates on context-aware Q&A from individual documents, and HotPotQA, which necessitates multi-hop reasoning across various documents.
They utilized a Llama-3.1-8B model featuring a 128,000-token context window. For RAG, they paired the LLM with two retrieval systems to extract passages relevant to the inquiry: the fundamental BM25 algorithm and OpenAI embeddings. For CAG, they embedded multiple documents from the benchmark into the prompt, permitting the model to determine which passages to employ in answering the question. Their experiments demonstrate that CAG surpassed both RAG systems in the majority of scenarios.
Final Thoughts: CAG’s Promise and Considerations
CAG surpasses both sparse RAG (BM25 retrieval) and dense RAG (OpenAI embeddings) (source: arXiv)
“By loading the complete context from the test set, our system eradicates retrieval inaccuracies and guarantees comprehensive reasoning across all pertinent information,” the scholars report. “This benefit is particularly noticeable in situations where RAG systems may fetch incomplete or irrelevant excerpts, resulting in subpar answer formulation.”
CAG also considerably shortens the duration needed to produce the answer, especially as the length of the reference text increases.

Nevertheless, CAG should not be perceived as a panacea and must be applied with prudence. It is particularly suited for situations where the knowledge database remains relatively stable and is sufficiently small to fit within the model’s context capacity. Organizations should also be mindful of circumstances where their documents include conflicting facts based on their context, which might complicate the model during inference.
The most effective method to gauge whether CAG is appropriate for your specific application is to conduct several trials. Fortunately, the deployment of CAG is quite simple and should invariably be seen as a preliminary measure before committing to more development-heavy RAG alternatives.
1 Trackback / Pingback