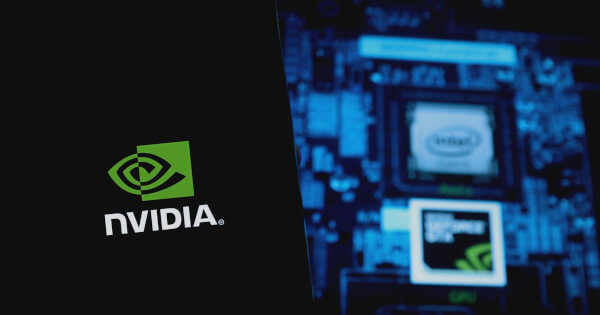
Jan 10, 2025 14:13
NVIDIA unveils Nemotron-CC, a 6.3-trillion-token English dataset, improving pretraining for substantial language models with pioneering data organization techniques.
NVIDIA has revealed the launch of Nemotron-CC, an innovative 6.3-trillion-token English dataset aimed at enhancing the pretraining of large language models (LLMs). This dataset, sourced from Common Crawl, aspires to boost the precision and effectiveness of LLMs through pioneering data organization methods, including the incorporation of 1.9 trillion tokens of synthetically generated content, as per NVIDIA.
Advancing LLM Pretraining
NVIDIA’s project responds to a significant demand in LLM training, where the caliber of pretraining datasets is crucial. While contemporary models like Meta’s Llama series have relied on datasets encompassing up to 15 trillion tokens, the precise makeup of these datasets is predominantly undisclosed. Nemotron-CC aims to bridge this gap by offering the broader community a high-quality dataset capable of facilitating both short and extended token horizon training.
Conventional datasets frequently forfeit up to 90% of data to enhance benchmark accuracies, limiting their practicality for extensive training. Nemotron-CC, however, illustrates how to convert Common Crawl data into a superior dataset, surpassing even the Llama 3.1 8B model through sophisticated techniques such as classifier ensembling and synthetic data rewording.
Notable Achievements
The effectiveness of Nemotron-CC is demonstrated by its results in various benchmarks. When training 8B parameter models with one trillion tokens, the high-quality subset Nemotron-CC-HQ outshines leading datasets like DCLM, raising MMLU scores by 5.6 points. Additionally, the full 6.3-trillion-token dataset matches DCLM on MMLU while providing four times more distinctive real tokens. This facilitates efficient training over long token horizons, with Nemotron-CC-trained models exceeding Llama 3.1 8B in various metrics, including a 5-point enhancement in MMLU and a 3.1-point uplift in ARC-Challenge scores.
Pioneering Data Organization Techniques
The creation of Nemotron-CC involved several crucial insights. By combining different model-based classifiers, NVIDIA was capable of selecting a wider selection of high-quality tokens. Furthermore, rephrasing strategies minimized noise and errors, producing diverse and precious data variations. The choice to deactivate traditional heuristic filters further elevated the dataset’s quality without sacrificing accuracy.
NVIDIA employed its NeMo Curator tool to extract and enhance data from Common Crawl, applying filters for language, deduplication, and quality classification. This approach was supported by synthetic data generation, contributing about two trillion tokens to the dataset.
Future Opportunities
Nemotron-CC is positioned as an essential asset for pretraining advanced LLMs across different token horizons. NVIDIA intends to broaden its offerings by launching additional specialized datasets, including those concentrated on particular areas such as mathematics, to further augment LLM abilities.
Image source: Shutterstock
Be the first to comment