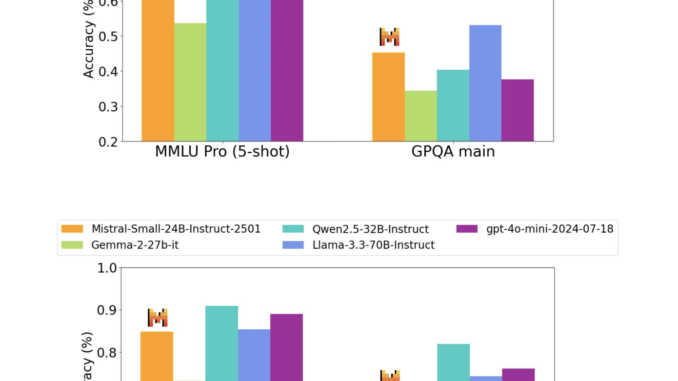
Creating compact yet high-efficiency language models presents a notable obstacle within artificial intelligence. Extensive models frequently necessitate significant computational resources, rendering them unattainable for numerous users and organizations with restricted hardware capacities. Furthermore, there is an increasing need for techniques that can tackle a variety of tasks, facilitate multilingual exchange, and deliver precise responses efficiently without compromising quality. Striking a balance between performance, scalability, and accessibility is essential, especially for promoting local implementations and safeguarding data privacy. This underscores the necessity for inventive strategies to develop smaller, resource-efficient models that offer capabilities akin to their larger equivalents while remaining adaptive and economical.
Recent progress in natural language processing has concentrated on crafting large-scale models, including GPT-4, Llama 3, and Qwen 2.5, which exhibit outstanding performance across a multitude of tasks but require considerable computational power. Initiatives to establish smaller, more efficient models involve instruction-tuned systems and quantization methods, allowing for local use while preserving competitive performance. Multilingual models such as Gemma-2 have elevated language comprehension in various fields, while advancements in function invocation and expanded context lengths have enhanced task-specific flexibility. Despite these advancements, achieving a harmony between performance, efficiency, and accessibility remains pivotal in the development of smaller, high-caliber language models.
Mistral AI has introduced the Small 3 (Mistral-Small-24B-Instruct-2501) model. This is a compact yet robust language model crafted to deliver cutting-edge performance with just 24 billion parameters. Fine-tuned on a variety of instruction-based tasks, it achieves superior reasoning, multilingual capabilities, and seamless application integration. In contrast to larger models, Mistral-Small is optimized for effective local deployment, accommodating devices such as RTX 4090 GPUs or laptops with 32GB RAM through quantization. With a 32k context window, it excels at managing extensive input while maintaining quick responsiveness. The model also features elements like JSON-based output and native function calling, enhancing its versatility for conversational and task-focused implementations.
To accommodate both commercial and non-commercial uses, the approach is made open-source under the Apache 2.0 license, guaranteeing flexibility for developers. Its sophisticated architecture ensures low latency and rapid inference, appealing to businesses and hobbyists alike. The Mistral-Small model prioritizes accessibility without sacrificing quality, bridging the divide between large-scale performance and resource-efficient deployment. By overcoming primary challenges in scalability and efficiency, it establishes a benchmark for compact models, competing with the performance of larger systems such as Llama 3.3-70B and GPT-4o-mini while being considerably easier to integrate into affordable setups.
The Mistral-Small-24B-Instruct-2501 model exhibits remarkable performance across numerous benchmarks, matching or surpassing larger models like Llama 3.3-70B and GPT-4o-mini in specific assignments. It achieves high accuracy in reasoning, multilingual processing, and coding benchmarks, scoring 84.8% on HumanEval and 70.6% on math tasks. With a 32k context window, the model adeptly manages extensive input, ensuring strong instruction-following capabilities. Evaluations underscore its outstanding performance in instruction adherence, conversational reasoning, and multilingual comprehension, securing competitive scores on both public and proprietary datasets. These findings highlight its efficiency, making it a viable alternative to larger models for a wide range of applications.
In summary, The Mistral-Small-24B-Instruct-2501 establishes a new benchmark for efficiency and performance in smaller-scale large language models. With 24 billion parameters, it provides outstanding outcomes in reasoning, multilingual comprehension, and coding tasks comparable to larger models while maintaining resource efficiency. Its 32k context window, finely-tuned instruction-following skills, and compatibility with local deployment make it ideal for various applications, spanning from conversational agents to domain-specific tasks. The model’s open-source nature under the Apache 2.0 license enhances its accessibility and adaptability. Mistral-Small-24B-Instruct-2501 signifies a substantial advancement toward developing powerful, compact, and versatile AI solutions for both community and enterprise applications.
Explore the Technical Details, mistralai/Mistral-Small-24B-Instruct-2501, and mistralai/Mistral-Small-24B-Base-2501. All appreciation for this research goes to the project’s researchers. Additionally, don’t forget to follow us on Twitter and join our Telegram Channel and LinkedIn Group. Also, remember to become part of our 70k+ ML SubReddit.
🚨 Meet IntellAgent: An Open-Source Multi-Agent Framework to Evaluate Complex Conversational AI System (Promoted)
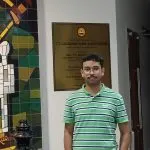
Sana Hassan, an intern in consulting at Marktechpost and a student pursuing dual degrees at IIT Madras, exhibits a fervor for utilizing technology and AI to tackle genuine global issues. With a strong desire to resolve real-world problems, he contributes an innovative outlook at the conjunction of AI and practical solutions.
1 Trackback / Pingback