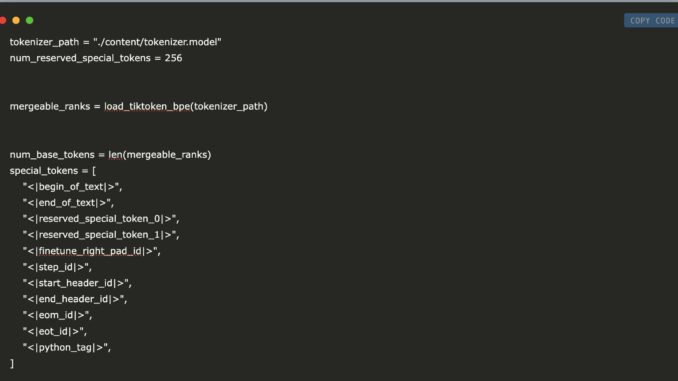
Tiktoken: Python Guide for NLP Enthusiasts
In this guide, we will explore how to build a personalized tokenizer utilizing the tiktoken library. This method entails loading a pre-trained tokenizer model, establishing both base and distinctive tokens, commencing the tokenizer with a designated regular expression for token separation, and validating its performance through encoding and decoding a few sample texts. This configuration is vital for NLP assignments that necessitate precise management over text tokenization.
import tiktoken
from tiktoken.load import load_tiktoken_bpe
import json
Here, we recruit multiple libraries critical for text analysis and machine learning. It employs Path from pathlib to simplify file path handling, while tiktoken and load_tiktoken_bpe assist in loading and utilizing a Byte Pair Encoding tokenizer.
num_reserved_special_tokens = 256mergeable_ranks = load_tiktoken_bpe(tokenizer_path)num_base_tokens = len(mergeable_ranks)
special_tokens = [
“<|begin_of_text|>”,
“<|end_of_text|>”,
“<|reserved_special_token_0|>”,
“<|reserved_special_token_1|>”,
“<|finetune_right_pad_id|>”,
“<|step_id|>”,
“<|start_header_id|>”,
“<|end_header_id|>”,
“<|eom_id|>”,
“<|eot_id|>”,
“<|python_tag|>”,
]
In this section, we determine the path to the tokenizer model and specify 256 reserved unique tokens. Next, it loads the mergeable ranks that constitute the base vocabulary, computes the number of base tokens, and outlines a list of special tokens for indicating text boundaries and various reserved functions.
f”<|reserved_special_token_{2 + i}|>”
for i in range(num_reserved_special_tokens – len(special_tokens))
]
special_tokens = special_tokens + reserved_tokenstokenizer = tiktoken.Encoding(
name=Path(tokenizer_path).name,
pat_str=r”(?i:’s|’t|’re|’ve|’m|’ll|’d)|[^rnp{L}p{N}]?p{L}+|p{N}{1,3}| ?[^sp{L}p{N}]+[rn]*|s*[rn]+|s+(?!S)|s+”,
mergeable_ranks=mergeable_ranks,
special_tokens={token: len(mergeable_ranks) + i for i, token in enumerate(special_tokens)},
)
At this point, we dynamically generate additional reserved tokens to achieve a total of 256, subsequently attaching them to the existing special tokens list. It establishes the tokenizer with tiktoken, encoding text using a specified regular expression for separation and employing the loaded mergeable ranks as the foundational vocabulary while associating special tokens with unique token identifiers.
# Validate the tokenizer with a sample sentence
#————————————————————————-
sample_text = “Hello, this is a test of the updated tokenizer!”
encoded = tokenizer.encode(sample_text)
decoded = tokenizer.decode(encoded)print(“Sample Text:”, sample_text)
print(“Encoded Tokens:”, encoded)
print(“Decoded Text:”, decoded)
We assess the tokenizer by transforming a sample sentence into token identifiers and subsequently decoding those identifiers back into textual format. It displays the original text, encoded tokens, and the decoded text to verify that the tokenizer functions accurately.
In this instance, we encode the phrase “Hey” into its equivalent token IDs by utilizing the tokenizer’s encoding function.
To summarize, following this guide will equip you with the knowledge to establish a custom BPE tokenizer employing the TikToken library. You learned how to load a pre-trained tokenizer model, designate both base and distinct tokens, and launch the tokenizer using a specific regular expression for token division. Ultimately, you confirmed the tokenizer’s operation by encoding and decoding sample texts. This configuration serves as a fundamental phase for any NLP endeavor that demands personalized text analysis and tokenization.
Here is the Colab Notebook corresponding to the aforementioned project. Additionally, don’t forget to follow us on Twitter and become a member of our Telegram Channel and LinkedIn Group. Don’t overlook the opportunity to join our 75k+ ML SubReddit.
🚨 Recommended Open-Source AI Platform: ‘IntellAgent is an Open-Source Multi-Agent Framework to Evaluate Complex Conversational AI System’ (Promoted)
Asif Razzaq serves as the CEO of Marktechpost Media Inc. Being a forward-thinking entrepreneur and engineer, Asif is dedicated to utilizing the power of Artificial Intelligence for societal benefits. His latest venture is the establishment of an Artificial Intelligence Media Platform, Marktechpost, notable for its comprehensive reporting on machine learning and deep learning news that is both technologically robust and easily comprehensible to a broad audience. The platform enjoys over 2 million monthly views, attesting to its widespread appeal among viewers.
Be the first to comment