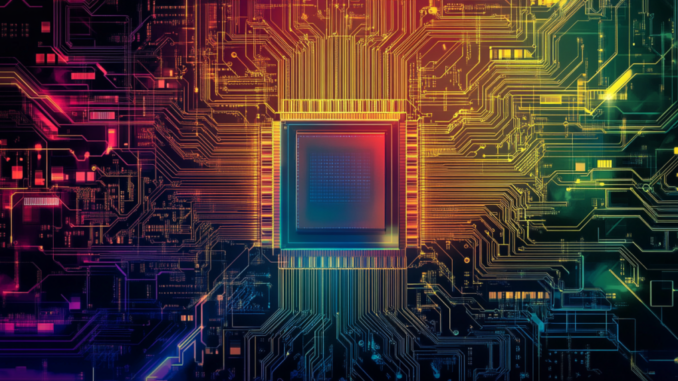
Subscribe to our daily and weekly newsletters for the most recent information and exclusive content on top-tier AI coverage. Discover More
On Wednesday, Google Cloud introduced its seventh-generation Tensor Processing Unit (TPU) named Ironwood, a customized AI accelerator which the firm asserts delivers over 24 times the processing capability of the fastest supercomputer globally when utilized at scale.
This new chip, revealed at Google Cloud Next ’25, marks a considerable shift in Google’s decade-long strategy for AI chip development. While earlier versions of TPUs were mainly crafted for both training and inference tasks, Ironwood is the inaugural model specifically designed for inference — the operation of employing trained AI models to generate predictions or responses.
“Ironwood is tailored to support this next chapter of generative AI and its immense computational and communication demands,” stated Amin Vahdat, Google’s Vice President and General Manager of ML, Systems, and Cloud AI, during a virtual press briefing prior to the event. “What we refer to as the ‘age of inference’ is upon us, where AI agents will actively gather and produce data to collaboratively provide insights and answers, beyond merely delivering data.”
Breaking computational limitations: Inside Ironwood’s 42.5 exaflops of AI power
The technical traits of Ironwood are impressive. When expanded to 9,216 chips per pod, Ironwood offers 42.5 exaflops of computational capacity — overshadowing El Capitan‘s 1.7 exaflops, which currently holds the title of the world’s fastest supercomputer. Each individual Ironwood chip achieves peak performance levels of 4,614 teraflops.
Ironwood also provides significant enhancements in memory and bandwidth. Each chip is equipped with 192GB of High Bandwidth Memory (HBM), six times that of Trillium, Google’s previous TPU introduced last year. Memory bandwidth reaches 7.2 terabits per second per chip, showcasing a 4.5x enhancement over Trillium.
Arguably most crucial in a period of power-restricted data centers, Ironwood offers double the performance per watt relative to Trillium and is nearly 30 times more power efficient than Google’s initial Cloud TPU from 2018.
“As available power becomes a limiting factor for delivering AI functionalities, we ensure significantly greater capacity per watt for our customers’ workloads,” Vahdat elaborated.
From model construction to ‘thinking machines’: The significance of Google’s inference focus today
The focus on inference instead of training symbolizes a pivotal turning point in the AI landscape. For years, the industry has concentrated on developing increasingly vast foundational models, with companies competing mainly over parameter size and training capabilities. Google’s shift towards optimizing inference indicates that we are transitioning into a new era where deployment efficiency and reasoning abilities become primary concerns.
This evolution is logical. Training occurs once, but inference actions happen billions of times each day as users interact with AI systems. The economics of AI are progressively linked to inference expenses, particularly as models become more intricate and computationally demanding.
During the press briefing, Vahdat disclosed that Google has seen a tenfold year-over-year surge in AI compute demand over the previous eight years — an astonishing total increase of 100 million. No current advancements from Moore’s Law could achieve this growth trajectory without specialized designs like Ironwood.
What stands out is the emphasis on “thinking models” that undertake complex reasoning processes rather than mere pattern recognition. This indicates that Google envisions the future of AI not only in larger models but in those capable of dissecting problems, reasoning through several stages, and imitating human-like cognitive processes.
Gemini’s cognitive engine: How Google’s next-gen models utilize advanced hardware
Google is establishing Ironwood as the cornerstone for its most sophisticated AI models, including Gemini 2.5, which the company touts as having “inherent thinking capacities.”
At the conference, also introduced Gemini 2.5 Flash, a more cost-efficient variant of its flagship model that “modulates the depth of reasoning according to the complexity of the prompt.” While Gemini 2.5 Pro is intended for intricate applications such as drug discovery and financial modeling, Gemini 2.5 Flash is aimed at everyday uses where responsiveness is vital.
The firm also showcased its complete range of generative media models, including text-to-image, text-to-video, and a newly launched text-to-music feature called Lyria. A demonstration illustrated how these tools could work in conjunction to produce a full promotional video for an event.
Beyond silicon: Google’s extensive infrastructure strategy encompasses network and software
Ironwood is merely one component of Google’s expansive AI infrastructure strategy. The company also revealed Cloud WAN, a managed wide-area network service allowing businesses access to Google’s planet-scale private network framework.
“Cloud WAN is a fully managed, efficient, and secure enterprise networking backbone that enhances network performance by up to 40%, while simultaneously lowering the total cost of ownership by the same margin,” Vahdat remarked.
Google is also broadening its software offerings for AI tasks, including Pathways, its machine learning runtime developed by Google DeepMind. Pathways on Google Cloud enables customers to expand model serving across numerous TPUs.
AI economics: How Google’s $12 billion cloud enterprise intends to prevail in the efficiency contest
These hardware and software announcements emerge at a critical juncture for Google Cloud, which reported $12 billion in revenue for Q4 2024, an increase of 30% year over year, according to its latest earnings statement.
The economics surrounding AI deployment are increasingly becoming a distinguishing factor in the cloud competition. Google faces fierce rivalry from Microsoft Azure, which has harnessed its partnership with OpenAI to carve out a strong market positioning, and Amazon Web Services, which continues to broaden its offerings in Trainium and Inferentia chips.
What differentiates Google’s strategy is its vertical integration. While competitors have collaborations with chip manufacturers or have acquired startups, Google has been producing TPUs in-house for over a decade. This grants the company unmatched control over its AI ecosystem, spanning from silicon to software to services.
By offering this technology to enterprise clients, Google is betting that its hard-earned expertise in developing chips for Search, Gmail, and YouTube will yield competitive advantages in the business market. The strategy is evident: provide the same infrastructure that drives Google’s own AI at scale to anyone willing to invest in it.
The multi-agent ecosystem: Google’s ambitious plan for AI systems to collaborate
Beyond hardware, Google laid out a vision for AI focused on multi-agent systems. The company introduced an Agent Development Kit (ADK) enabling developers to create systems wherein multiple AI agents can collaborate.
Perhaps
Most importantly, Google unveiled an “agent-to-agent interoperability protocol” (A2A), which allows AI agents developed on various frameworks and by diverse vendors to interact with one another.
“The year 2025 will serve as a transformative period where generative AI evolves from responding to individual inquiries to addressing intricate challenges via agent-based systems,” Vahdat forecasted.
Google is collaborating with more than 50 leading firms, such as Salesforce, ServiceNow, and SAP, to propel this interoperability standard forward.
Enterprise reality assessment: What Ironwood’s capabilities and efficiency signify for your AI strategy
For businesses implementing AI, these announcements could greatly lower the expenses and complications of operating advanced AI models. Ironwood’s enhanced efficiency may render the use of sophisticated reasoning models more cost-effective, while the agent interoperability protocol could assist enterprises in avoiding dependency on specific vendors.
The tangible effects of these innovations should not be underestimated. Numerous organizations have hesitated to deploy advanced AI models due to excessive infrastructure expenditures and energy usage. If Google can fulfill its performance-per-watt commitments, we could witness a fresh wave of AI adoption in sectors that have so far observed from the sidelines.
The multi-agent methodology is also crucial for corporations inundated by the complexities of deploying AI across various systems and vendors. By standardizing the communication protocols for AI systems, Google is striving to dismantle the barriers that have hindered AI’s influence in enterprises.
During the press conference, Google reiterated that it would share over 400 customer stories at Next ’25, illustrating the real business effects of its AI innovations.
The silicon arms race: Can Google’s customized chips and open standards redefine AI’s future?
As AI progresses, the infrastructure that supports it will grow increasingly vital. Google’s investments in specialized hardware such as Ironwood, alongside its agent interoperability efforts, indicate that the company is preparing for a future in which AI becomes more decentralized, intricate, and ingrained within business operations.
“Prominent models like Gemini 2.5 and the Nobel Prize-winning AlphaFold are all currently powered by TPUs,” Vahdat remarked. “With Ironwood, we are eager to see the AI advancements stimulated by our developers and Google Cloud clients when it launches later this year.”
The strategic consequences extend beyond Google’s own enterprise. By advocating for open standards in agent communication while retaining proprietary benefits in hardware, Google is engaged in a careful balancing act. The company aspires to see a thriving ecosystem (with Google infrastructure at its foundation), while still ensuring competitive differentiation.
The speed at which rivals react to Google’s hardware innovations, and whether the industry unifies around the suggested agent interoperability standards, will be critical elements to observe in the upcoming months. If history serves as a guide, we can anticipate Microsoft and Amazon to respond with their own optimization strategies for inference, potentially igniting a three-way contest to establish the most efficient AI infrastructure framework.
Be the first to comment