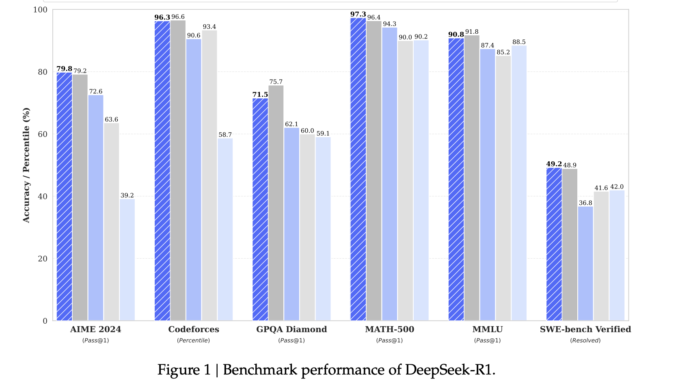
Extensive Language Models (ELMs) have achieved remarkable advancements in linguistic processing, excelling in activities such as comprehension, generation, and logical reasoning. Nevertheless, obstacles persist. Attaining resilient reasoning often necessitates considerable supervised fine-tuning, which constrains scalability and generalization. Additionally, challenges like inadequate readability and the need to balance computational efficiency with reasoning intricacy continue to exist, leading researchers to investigate alternative methods.
DeepSeek-R1: A Novel Method in LLM Reasoning
DeepSeek-AI’s latest research presents DeepSeek-R1, a model created to elevate reasoning capabilities via reinforcement learning (RL). This initiative led to the development of two models:
DeepSeek-R1-Zero, which is exclusively trained using RL and exhibits emergent reasoning traits, such as extended Chain-of-Thought (CoT) reasoning.
DeepSeek-R1, which expands upon its predecessor by integrating a multi-phase training framework, tackling issues like readability and language mixing while achieving high reasoning efficiency.
These models strive to bridge existing gaps, merging cutting-edge RL strategies with systematic training techniques to enhance scalability and adaptability.
Technological Innovations and Advantages
1. Reinforcement Learning for Reasoning Activities: DeepSeek-R1-Zero utilizes RL without dependence on supervised data. By employing Group Relative Policy Optimization (GRPO), it enhances reasoning by assessing several outputs, greatly boosting benchmark outcomes. For instance, its AIME 2024 pass@1 score increased from 15.6% to 71.0% during training.
2. Multi-Phase Training in DeepSeek-R1: DeepSeek-R1 integrates cold-start data—thousands of carefully selected CoT examples—to refine its foundational model prior to engaging in reasoning-centric RL. This mechanism guarantees that outputs maintain coherence and user-friendliness by incorporating rewards for language consistency.
3. Distillation for Minimized Models: To tackle computational limitations, DeepSeek-AI distilled six smaller models (ranging from 1.5B to 70B parameters) from DeepSeek-R1 utilizing Qwen and Llama frameworks. These models preserve robust reasoning capabilities, with the 14B distilled version attaining a pass@1 score of 69.7% on AIME 2024, exceeding the performance of certain larger models.
Results: Performance Observations
DeepSeek-R1’s performance is substantiated by benchmark findings:
Reasoning Benchmarks:
AIME 2024: 79.8% pass@1, outstripping OpenAI’s o1-mini.
MATH-500: 97.3% pass@1, analogous to OpenAI-o1-1217.
GPQA Diamond: 71.5% pass@1, excelling in fact-driven reasoning.
Coding and STEM Tasks:
Codeforces Elo rating: 2029, surpassing 96.3% of human contenders.
SWE-Bench Verified: 49.2% solution rate, competitive with other foremost models.
General Abilities:
Strong generalization capabilities were demonstrated on ArenaHard and AlpacaEval 2.0 benchmarks, achieving 92.3% and 87.6% victory rates, respectively.
Distilled Model Highlights: Smaller models such as DeepSeek-R1-Distill-Qwen-32B display considerable performance, with a pass@1 score of 72.6% on AIME 2024, illustrating effective scalability and practicality.

Conclusion: Enhancing Reasoning in AI
DeepSeek-AI’s DeepSeek-R1 and DeepSeek-R1-Zero signify substantial progress in reasoning abilities for LLMs. By utilizing RL, cold-start datasets, and distillation methodologies, these models tackle pivotal restrictions while fostering accessibility through open-source distribution under the MIT License. The API (‘model=deepseek-reasoner’) significantly boosts functionality for developers and researchers.
Looking forward, DeepSeek-AI intends to enhance multilingual support, upgrade software engineering features, and heighten prompt sensitivity. These initiatives are aimed at solidifying DeepSeek-R1 as a reliable option for reasoning-centric AI applications. By integrating deliberate training frameworks, DeepSeek-R1 showcases how AI can evolve to confront increasingly intricate challenges.
Explore the Paper, DeepSeek R1 and DeepSeek R1 Zero. All recognition for this investigation belongs to the researchers of this initiative. Additionally, don’t forget to follow us on Twitter and join our Telegram Channel and LinkedIn Group. Remember to join our 65k+ ML SubReddit.
🚨 [Recommended Read] Nebius AI Studio broadens its offerings with vision models, new language models, embeddings, and LoRA (Promoted)
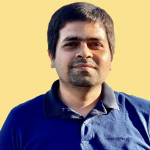
Asif Razzaq serves as the CEO of Marktechpost Media Inc. A forward-thinking entrepreneur and engineer, Asif is dedicated to utilizing the power of Artificial Intelligence for societal benefit. His latest undertaking is the launch of an Artificial Intelligence Media Platform, Marktechpost, recognized for its comprehensive coverage of machine learning and deep learning news that is both technically proficient and accessible to a broad audience. The platform has garnered over 2 million monthly views, demonstrating its appeal to viewers.
Be the first to comment