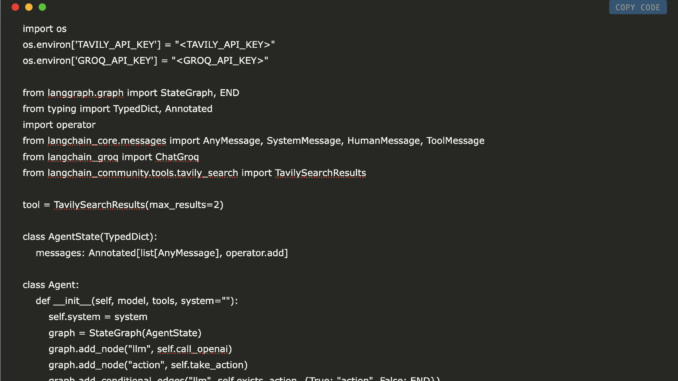
In our prior lesson, we created an AI agent capable of responding to inquiries by browsing the internet. Nevertheless, when constructing agents for extended tasks, two essential principles come into focus: durability and streaming. Durability allows you to preserve the status of an AI agent at any given moment, permitting you to continue from that status in later interactions. This is vital for applications that require long durations. Conversely, streaming enables you to convey real-time notifications about what the agent is engaged in at any instant, granting visibility and oversight over its operations. In this lesson, we’ll enrich our agent by incorporating these robust functionalities.
Establishing the AI Agent
Let’s begin by recreating our agent. We’ll load the essential environment variables, set up and import the necessary libraries, configure the Tavily search utility, define the AI agent’s status, and ultimately, construct the AI agent.
os.environ[‘TAVILY_API_KEY’] = “<TAVILY_API_KEY>”
os.environ[‘GROQ_API_KEY’] = “<GROQ_API_KEY>”from langgraph.graph import StateGraph, END
from typing import TypedDict, Annotated
import operator
from langchain_core.messages import AnyMessage, SystemMessage, HumanMessage, ToolMessage
from langchain_groq import ChatGroq
from langchain_community.tools.tavily_search import TavilySearchResultstool = TavilySearchResults(max_results=2)
class AgentState(TypedDict):
messages: Annotated[list[AnyMessage], operator.add]
class Agent:
def __init__(self, model, tools, system=””):
self.system = system
graph = StateGraph(AgentState)
graph.add_node(“llm”, self.call_openai)
graph.add_node(“action”, self.take_action)
graph.add_conditional_edges(“llm”, self.exists_action, {True: “action”, False: END})
graph.add_edge(“action”, “llm”)
graph.set_entry_point(“llm”)
self.graph = graph.compile()
self.tools = {t.name: t for t in tools}
self.model = model.bind_tools(tools)
def call_openai(self, state: AgentState):
messages = state[‘messages’]
if self.system:
messages = [SystemMessage(content=self.system)] + messages
message = self.model.invoke(messages)
return {‘messages’: [message]}
def exists_action(self, state: AgentState):
result = state[‘messages’][-1]
return len(result.tool_calls) > 0
def take_action(self, state: AgentState):
tool_calls = state[‘messages’][-1].tool_calls
results = []
for t in tool_calls:
print(f”Calling: {t}”)
result = self.tools[t[‘name’]].invoke(t[‘args’])
results.append(ToolMessage(tool_call_id=t[‘id’], name=t[‘name’], content=str(result)))
print(“Returning to the model!”)
return {‘messages’: results}
Incorporating Durability
To introduce durability, we’ll utilize LangGraph’s checkpointing capability. A checkpointer preserves the state of the agent following and between each node. Throughout this lesson, we’ll apply SqliteSaver, a straightforward checkpointer that utilizes SQLite, a built-in database. While we’ll implement an in-memory database for ease, you can effortlessly link it to an external database or utilize other checkpoint mechanisms like Redis or Postgres for enhanced durability.
import sqlite3
sqlite_conn = sqlite3.connect(“checkpoints.sqlite”,check_same_thread=False)
memory = SqliteSaver(sqlite_conn)
Subsequently, we’ll adjust our agent to accommodate a checkpointer:
def __init__(self, model, tools, checkpointer, system=””):
# All other elements remain unchanged
self.graph = graph.compile(checkpointer=checkpointer)
# All remaining components beyond this point stay the same
Now, we can instantiate our AI agent with durability enabled:
You may make multiple calls (either collectively or sequentially). \
Only seek out information when you are certain of what you want. \
If you need to research some details before posing a follow-up question, feel free to do so!
“””
model = ChatGroq(model=”Llama-3.3-70b-Specdec”)
bot = Agent(model, [tool], system=prompt, checkpointer=memory)
Incorporating Streaming
Streaming is pivotal for real-time updates. We will concentrate on two forms of streaming:
1. Streaming Messages: Emitting intermediate messages such as AI decisions and tool outcomes.
2. Streaming Tokens: Sending individual tokens from the LLM’s response. Let’s initiate by streaming messages. We will generate a human message and employ the stream method to witness the agent’s activities in real time.
thread = {“configurable”: {“thread_id”: “1”}}
for event in bot.graph.stream({“messages”: messages}, thread):
for v in event.values():
print(v[‘messages’])
Final output: The current weather in Texas is sunny with a temperature of 19.4°C (66.9°F) and a wind speed of 4.3 mph (6.8 kph)…..
When executing this, you’ll witness a flow of results. Initially, an AI message directs the agent to call Tavily, succeeded by a tool message containing the search findings, and finally, an AI message addressing the inquiry.
Comprehending Thread IDs
The thread_id is a significant aspect of the thread configuration. It enables the AI agent to manage distinct dialogues with various users or contexts. By designating a unique thread_id to each conversation, the AI agent can monitor multiple interactions simultaneously without confusion.
For instance, let’s carry on the conversation by asking, “What about in LA?” using the same thread_id:
thread = {“configurable”: {“thread_id”: “1”}}
for event in bot.graph.stream({“messages”: messages}, thread):
for v in event.values():
print(v)
Final output: The current weather in Los Angeles is sunny with a temperature of 17.2°C (63.0°F) and a wind speed of 2.2 mph (3.6 kph) ….
The agent deduces that we’re inquiring about the weather, owing to durability. To confirm, let’s inquire, “Which one is warmer?”:
thread = {“configurable”: {“thread_id”: “1”}}
for event in bot.graph.stream({“messages”: messages}, thread):
for v in event.values():
print(v)
Final
output: Texas has a higher temperature than Los Angeles. The present temperature in Texas stands at 19.4°C (66.9°F), whereas the current temperature in Los Angeles is 17.2°C (63.0°F)The agent accurately assesses the weather conditions in Texas and LA. To examine whether persistence ensures interactions remain distinct, let’s pose the identical inquiry with an alternate thread_id:
thread = {“configurable”: {“thread_id”: “2”}}
for event in bot.graph.stream({“messages”: messages}, thread):
for v in event.values():
print(v)
Output: I require further details to respond to that inquiry. Could you kindly provide additional context or clarify the two items you are contrasting?
This time, the agent becomes bewildered as it lacks access to the previous conversation’s record.
Streaming Tokens
To stream tokens, we’ll utilize the astream_events method, which operates asynchronously. We’ll also transition to an async checkpointer.
from langgraph.checkpoint.sqlite.aio import AsyncSqliteSaver
async with AsyncSqliteSaver.from_conn_string(“:memory:”) as checkpointer:
abot = Agent(model, [tool], system=prompt, checkpointer=checkpointer)
messages = [HumanMessage(content=”What is the weather in SF?”)]
thread = {“configurable”: {“thread_id”: “4”}}
async for event in abot.graph.astream_events({“messages”: messages}, thread, version=”v1″):
kind = event[“event”]
if kind == “on_chat_model_stream”:
content = event[“data”][“chunk”].content
if content:
# An empty content in the context of OpenAI signifies
# that the model is requesting a tool to be activated.
# Thus, we only display non-empty content
print(content, end=”|”)
This method will stream tokens instantaneously, providing a real-time insight into the agent’s reasoning.
Conclusion
In the forthcoming tutorial, we will delve into human-in-the-loop engagements, where persistence is paramount for facilitating seamless cooperation between humans and AI agents. Stay tuned!
References:
(DeepLearning.ai) https://learn.deeplearning.ai/courses/ai-agents-in-langgraph
Also, be sure to follow us on Twitter and join our Telegram Channel along with our LinkedIn Group. Don’t forget to join our 75k+ ML SubReddit.
🚨 Meet IntellAgent: An Open-Source Multi-Agent Framework to Evaluate Complex Conversational AI Systems (Promoted)
Vineet Kumar is an intern consultant at MarktechPost. He is currently studying for his BS at the Indian Institute of Technology(IIT), Kanpur. He is passionate about Machine Learning and is enthusiastic about research and the latest breakthroughs in Deep Learning, Computer Vision, and associated domains.
1 Trackback / Pingback