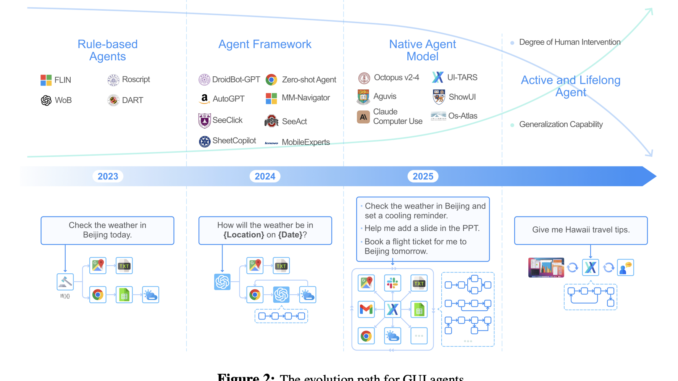
GUI agents endeavor to accomplish genuine tasks in digital atmospheres by interpreting and engaging with graphical interfaces like buttons and text fields. The most substantial open issues are found in empowering agents to analyze intricate, changing interfaces, devise effective strategies, and carry out precise actions, including locating clickable areas or completing text fields. Furthermore, these agents necessitate memory mechanisms to remember previous actions and adjust to fresh situations. A crucial issue confronting contemporary, consolidated end-to-end models is the lack of integrated perception, reasoning, and action within fluid workflows that utilize high-quality data embodying this expansive vision. In the absence of such data, these systems struggle to adapt to a variety of dynamic environments and scale effectively.
Present strategies for GUI agents predominantly rely on rules and are greatly contingent on predefined regulations, frameworks, and human engagement, making them neither adaptable nor scalable. Rule-based agents, such as Robotic Process Automation (RPA), function within structured settings using human-established heuristics and demand direct system access, rendering them ineffective for dynamic or limited interfaces. Framework-based agents leverage foundational models like GPT-4 for multi-step reasoning yet still rely on manual processes, prompts, and external scripts. These techniques are fragile, require ongoing updates for changing tasks, and fall short of seamlessly integrating learning from real-world encounters. Native agent models aim to combine perception, reasoning, memory, and action under a single framework by minimizing human engineering via end-to-end training. However, these models remain dependent on curated data and training direction, thus restricting their flexibility. The current methods do not permit agents to learn independently, adjust effectively, or manage unpredictable situations without human input.

In response to the obstacles encountered in GUI agent development, researchers from ByteDance Seed and Tsinghua University have put forth the UI-TARS framework aimed at enhancing native GUI agent models. This framework merges advanced perception, cohesive action modeling, sophisticated reasoning, and iterative training, which assists in diminishing human involvement while improving generalization. It allows for a comprehensive comprehension with accurate labeling of interface components utilizing an extensive dataset of GUI screenshots. This ushers in a standardized action space to normalize platform interactions and employs vast action traces to enhance multi-step execution. The framework further incorporates System-2 reasoning for intentional decision-making and continuously sharpens its abilities through online interaction traces.

Scientists formulated the framework utilizing a number of essential tenets. Improved perception was harnessed to guarantee that GUI components are accurately identified through selected datasets for tasks such as element identification and comprehensive captioning. Integrated action modeling correlates the element descriptions with spatial coordinates to attain accurate grounding. System-2 reasoning was incorporated to embrace various logical architectures and explicit cognitive processes, steering intentional actions. It adopted iterative training for dynamic data accumulation and interaction enhancement, error identification, and adjustment through reflective tuning for resilient and scalable education with minimal human intervention.

Researchers assessed the UI-TARS which was trained on a collection of approximately 50B tokens across various dimensions, encompassing perception, grounding, and agent capabilities. The model was created in three forms: UI-TARS-2B, UI-TARS-7B, and UI-TARS-72B, alongside comprehensive experiments affirming their benefits. When compared to benchmarks such as GPT-4o and Claude-3.5, UI-TARS exhibited superior performance in evaluations of perception, including VisualWebBench and WebSRC. UI-TARS outshone models like UGround-V1-7B in grounding across numerous datasets, showcasing strong abilities in high-complexity situations. In terms of agent assignments, UI-TARS excelled in Multimodal Mind2Web and Android Control as well as environments like OSWorld and AndroidWorld. The findings underscored the significance of system-1 and system-2 reasoning, with system-2 reasoning proving advantageous in diverse, real-world contexts, although it necessitated multiple candidate outputs for ideal performance. Enlarging the model size enhanced reasoning and decision-making, especially in online tasks.


To sum up, the suggested approach, UI-TARS, enhances GUI automation by combining superior perception, unified action modeling, system-2 reasoning, and iterative training. It achieves top-tier performance, outperforming previous systems like Claude and GPT-4o, and adeptly manages intricate GUI tasks with minimal human input. This contribution establishes a solid foundation for forthcoming studies, especially in active and lifelong learning domains, where agents can independently develop through ongoing real-world engagement, setting the stage for additional progress in GUI automation.
Explore the Paper. All acknowledgments for this study belong to the researchers involved in this initiative. Additionally, don’t forget to follow us on Twitter and join our Telegram Channel and LinkedIn Group. Be sure to join our 70k+ ML SubReddit.
🚨 [Recommended Read] Nebius AI Studio broadens with vision models, fresh language models, embeddings, and LoRA (Promoted)
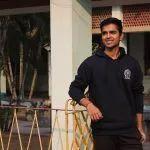
Divyesh is a consulting intern at Marktechpost. He is currently studying for a BTech in Agricultural and Food Engineering at the Indian Institute of Technology, Kharagpur. He possesses a keen interest in Data Science and Machine Learning, aiming to incorporate these cutting-edge technologies into the agricultural sector to tackle various challenges.
Be the first to comment