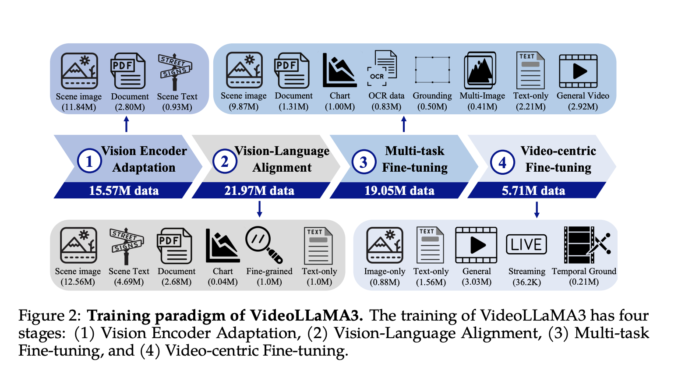
Progress in multimodal intelligence relies on the processing and comprehension of images and videos. Pictures can expose static settings by conveying information about elements such as objects, text, and spatial connections. Nevertheless, this process presents considerable challenges. Understanding video entails monitoring variations over time, among other tasks, while maintaining consistency throughout frames, which necessitates efficient content management and temporal connections. These activities become increasingly intricate since the gathering and labeling of video-text datasets are notably more complex than their image-text counterparts.
Conventional techniques for multimodal large language models (MLLMs) encounter difficulties in video comprehension. Strategies involving sparsely sampled frames, basic links, and image-centric encoders do not effectively capture temporal dependencies and dynamic content. Approaches like token compression and extended context windows struggle with the intricacies of long-form videos, and the integration of audio and visual inputs often lacks smooth interaction. Efforts in real-time processing and scaling model dimensions continue to be ineffective, and current structures fail to optimize for extended video tasks.
To tackle the challenges of video comprehension, researchers from Alibaba Group introduced the VideoLLaMA3 framework. This framework integrates Any-resolution Vision Tokenization (AVT) and Differential Frame Pruner (DiffFP). AVT enhances traditional fixed-resolution tokenization, allowing vision encoders to dynamically process variable resolutions and minimize information loss. This is accomplished by adapting ViT-based encoders with 2D-RoPE for flexible positioning embedding. To maintain critical information, DiffFP addresses redundant and lengthy video tokens by eliminating frames with minimal differences based on a 1-norm distance between patches. The dynamic resolution management, combined with effective token minimization, enhances representation while lowering costs.
The framework comprises a vision encoder, video compressor, projector, and large language model (LLM), initializing the vision encoder with a pre-trained SigLIP model. It extracts visual tokens, while the video compressor reduces the representation of video tokens. The projector connects the vision encoder to the LLM, utilizing Qwen2.5 models for the LLM. Training is carried out in four phases: Vision Encoder Adaptation, Vision-Language Alignment, Multi-task Fine-tuning, and Video-centric Fine-tuning. The initial three phases concentrate on image comprehension, while the concluding phase augments video understanding by incorporating temporal details. The Vision Encoder Adaptation Stage hones the vision encoder, initialized with SigLIP, on a substantial image dataset, enabling it to process images at varied resolutions. The Vision-Language Alignment Stage introduces multimodal knowledge, making the LLM and vision encoder trainable to assimilate vision and language comprehension. In the Multi-task Fine-tuning Stage, instruction fine-tuning is conducted using multimodal question-answering data, featuring image and video inquiries, enhancing the model’s proficiency in following natural language instructions and managing temporal details. The Video-centric Fine-tuning Stage unlocks all parameters to bolster the model’s video comprehension abilities. Training data is sourced from various materials such as scene images, documents, charts, fine-grained images, and video content, assuring a thorough multimodal understanding.
Researchers performed experiments to assess the efficacy of VideoLLaMA3 across image and video tasks. For image-centric tasks, the model was evaluated on document analysis, mathematical problem-solving, and multi-image comprehension, surpassing prior models, demonstrating enhancements in chart analysis and real-world knowledge question answering (QA). In video-centric tasks, VideoLLaMA3 excelled in benchmarks such as VideoMME and MVBench, showcasing its competency in general video comprehension, long-form video analysis, and temporal reasoning. The 2B and 7B models performed quite competitively, with the 7B model excelling in most video challenges, highlighting the model’s proficiency in multimodal tasks. Significant advancements were also reported in OCR, mathematical reasoning, multi-image understanding, and extended video comprehension.
Ultimately, the introduced framework enhances vision-centric multimodal models, providing a robust structure for analyzing images and videos. By leveraging high-quality image-text datasets, it overcomes challenges in video comprehension and temporal dynamics, achieving impressive results across benchmarks. Nonetheless, issues concerning the quality of video-text datasets and real-time processing persist. Future inquiries could improve video-text datasets, refine for real-time efficiency, and incorporate additional modalities like audio and speech. This work establishes a foundation for future progress in multimodal understanding, enhancing efficiency, generalization, and integration.
Check out the Paper and GitHub Page. All credit for this research goes to the researchers of this project. Also, don’t forget to follow us on Twitter and join our Telegram Channel and LinkedIn Group. Don’t forget to join our 70k+ ML SubReddit.
🚨 [Recommended Read] Nebius AI Studio expands with vision models, new language models, embeddings and LoRA (Promoted)
Be the first to comment