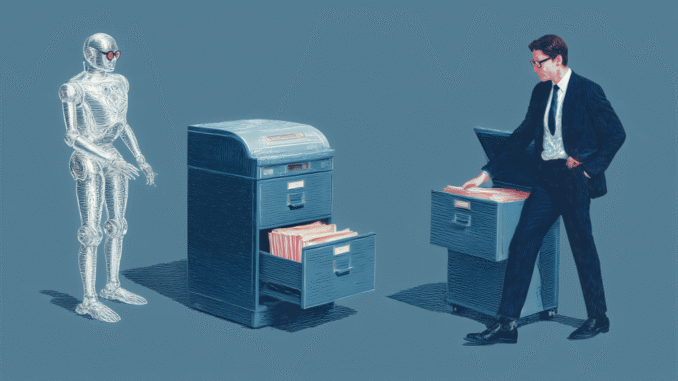
Subscribe to our daily and weekly newsletters for the most recent updates and exclusive material on cutting-edge AI reporting. Discover More
The French AI startup Pleias created a stir late last year with the introduction of its ethically developed Pleias 1.0 series of compact language models — among the earliest to be constructed entirely by scraping “open” data, defined as information explicitly designated as public domain, open source, or unlicensed and free from copyright restrictions.
Now, the company has revealed the rollout of two open source small-scale reasoning models crafted specifically for retrieval-augmented generation (RAG), citation synthesis, and structured multilingual outputs.
The release encompasses two primary models — Pleias-RAG-350M and Pleias-RAG-1B — each also offered in CPU-optimized GGUF format, resulting in a total of four deployable variants.
All models are grounded in Pleias 1.0 and can function autonomously or in combination with other LLMs that the organization may currently utilize or aspire to implement. All seem to be distributed under a permissive Apache 2.0 open source license; thus, organizations can adopt, modify, and deploy them for commercial purposes.
RAG, as you might recall, is the extensively utilized strategy that businesses and organizations can leverage to connect an AI large language model (LLM) such as OpenAI’s GPT-4o, Google’s Gemini 2.5 Flash, Anthropic’s Claude Sonnet 3.7, or Cohere’s Command-A, along with open-source options like Llama 4 and DeepSeek V3 to external knowledge repositories, which include enterprise documents and cloud storage systems.
This is often imperative for organizations aiming to develop chatbots and other AI applications that cite their internal guidelines or product listings (alternatively, prompting a lengthy context LLM with all necessary information might not be suitable for enterprise scenarios where security and costs per token are critical concerns).
The Pleias-RAG model family represents the latest initiative to bridge the divide between accuracy and efficiency in compact language models.
These models target enterprises, developers, and researchers seeking economical substitutes for expansive language models without sacrificing traceability, multilingual features, or structured reasoning processes.
The intended user base is primarily Pleias’s native continent of Europe, as co-founder Alexander Doria conveyed to VentureBeat through a direct message on the X social platform:
“A major impetus has been the challenges of scaling RAG applications in Europe. Most private organizations have limited GPUs (this might have changed, but not long ago less than 2% of all [Nvidia] H100 [GPUs] were located in Europe). Nonetheless, there are significant incentives to self-host for regulatory purposes, including GDPR.
“SLMs have advanced considerably over the past year; however, they are frequently conceived as ‘mini-chatbots,’ and we have noted a considerable decline in performance in languages other than English, both regarding comprehension and text generation quality. Thus, we are pleased to have achieved most of our goals:
An actual alternative to 7-8 billion parameter models for RAG even on CPU and other resource-constrained infrastructures.
Fully verifiable models equipped with citation support.
Retention of European language performance.”
However, the models being open source under the Apache 2.0 license implies anyone could adopt and utilize them freely worldwide.
Focused on grounding, citations, and facts
A significant attribute of the new Pleias-RAG models is their inherent support for source citation with direct quotes, seamlessly integrated into the model’s inference mechanism.
In contrast to post-hoc citation strategies or external chunking systems, the Pleias-RAG models generate citations inherently, employing a syntax derived from Wikipedia’s referencing style.
This methodology allows for more concise, easy-to-read citation snippets while ensuring verifiability.
Citation grounding plays a critical function in regulated environments.
For industries like healthcare, law, and finance — where decision-making requires thorough documentation and traceability — these integrated references provide a direct pathway to auditability. Pleias presents this design choice as an ethical necessity, aligning with the growing regulatory expectations for explainable AI.
Proto agentic?
The Pleias-RAG models are characterized as “proto-agentic” — capable of independently evaluating whether a query is comprehensible, determining if it is simple or intricate, and deciding whether to respond, reformulate, or decline based on source adequacy.
Their structured outputs encompass language detection, query and source analysis reports, along with a rational answer.
Despite their relatively modest size (Pleias-RAG-350M comprises just 350 million parameters), these models display behaviors traditionally linked to larger, agentic systems.
Pleias asserts that these functionalities arise from a specialized mid-training pipeline that combines synthetic data generation with iterative reasoning prompts.
Pleias-RAG-350M is specifically crafted for resource-limited environments. It performs effectively on standard CPUs, including mobile-class infrastructure.
According to internal benchmarks, the unquantized GGUF variant produces comprehensive reasoning outputs in approximately 20 seconds on setups with 8GB RAM. Its compact size places it in a niche market with very few rivals, such as Qwen-0.5 and SmolLM, but with a significantly stronger focus on structured source synthesis.
Competitive performance across tasks and languages
In evaluation benchmarks, Pleias-RAG-350M and Pleias-RAG-1B outperform most open-weight models under 4 billion parameters, including Llama-3.1-8B and Qwen-2.5-7B, on tasks such as HotPotQA, 2WikiMultiHopQA, and MuSiQue.
These multi-hop RAG benchmarks assess the model’s ability to reason across several documents and identify distractors — common prerequisites in enterprise-grade knowledge systems.
The models’ capabilities extend to multilingual contexts. On translated benchmark collections across French, German, Spanish, and Italian, the Pleias models exhibit minimal performance degradation.
This distinguishes them from other SLMs, which generally experience a performance decline of 10–35% when processing non-English queries.
The multilingual support results from meticulous tokenizer design and synthetic adversarial training that incorporates language-switching exercises. The models are adept at not only identifying the language of a user query but also responding in the same language—an essential feature for global applications.
Additionally, Doria emphasized how the models could enhance the performance of other existing models an enterprise might be utilizing:
“We envision the models used in orchestrated settings, especially considering their low computational costs. An intriguing outcome on the evaluation front: even the 350M model provided considerably different answers compared to responses generated by [Meta] Llama and [Alibaba] Qwen. Thus, there’s a genuine complementarity we attribute to our reasoning pipeline, extending beyond mere cost-effectiveness…”
Open access and licensing
According to Doria and a technical document outlining the training of the Pleias-RAG family, the models were trained on: “Common Corpus to form the RAG training set (all 3 million samples originated from it). We utilized [Google] Gemma additionally for generating reasoning synthetic traces since the license permitted reuse/retraining.”
Both models are released under the Apache 2.0 license, permitting commercial reuse.and incorporation into larger frameworks.
Pleias highlights the models’ appropriateness for integration into search-enhanced assistants, educational resources, and customer support systems. The firm also offers an API library to ease structured input-output formatting for developers.
The models’ introduction is part of a larger initiative by Pleias to redefine small LLMs as instruments for structured reasoning, rather than simply as versatile conversational bots.
By utilizing an external memory framework and systematic referencing techniques, the Pleias-RAG series provides a clear, verifiable alternative to less transparent cutting-edge models.
Prospective outlook
In the future, Pleias intends to enhance the models’ functionalities through extended context management, closer search integration, and personality calibration for a more uniform identity portrayal.
Reinforcement learning is also under investigation, especially in areas like citation precision, where quote verification can be assessed algorithmically.
The team is also actively collaborating with organizations like the Wikimedia Foundation to facilitate targeted search integrations utilizing reliable sources.
Eventually, the current application of RAG-specific implementations, models, and workflows may diminish as more sophisticated AI models are developed and implemented, those that inherently include RAG and agentic tool utilization. As Doria conveyed to VentureBeat via DM:
“In the long run, I believe that both traditional RAG pipelines and long-context models are destined to be disrupted by search agents. We have started advancing in this direction: that’s why the model already includes numerous features that are now externalized in RAG applications (query reformulation, reranking, etc.). We certainly aim to go beyond and incorporate search capabilities and source processing features directly within the model itself. I am convinced that RAG will fade away as it becomes automated by agentic models capable of orchestrating their own workflows.“
With Pleias-RAG-350M and 1B, the company is wagering that small models—when complemented by robust reasoning frameworks and verifiable outputs—can rival significantly larger counterparts, particularly in multilingual and resource-constrained environments.
Be the first to comment