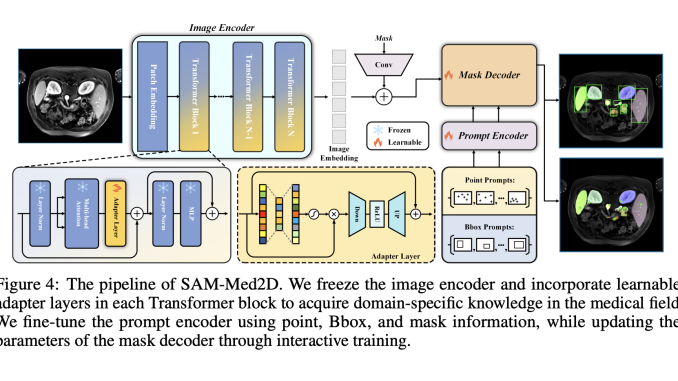
Medical AI image segmentation is crucial for analyzing medical imagery by identifying and distinguishing various tissues, organs, or areas of interest. With precise segmentation, healthcare professionals can accurately identify and locate diseased regions, leading to more reliable diagnosis and treatment. Moreover, comprehensive insights into the morphology, structure, and functionality of different tissues or organs are afforded through both quantitative and qualitative evaluations of medical images, facilitating disease investigation. However, many existing methodologies are limited to specific modalities, organs, or pathologies due to the unique characteristics of medical imaging, which include a diverse range of modalities, intricate tissue and organ structures, and a lack of annotated data.
This limitation makes algorithms challenging to generalize and adapt for different clinical applications. Recently, the AI community has shown a growing interest in the development of large-scale models. Innovations in general AI models such as ChatGPT2, ERNIE Bot 3, DINO, SegGPT, and SAM have made it feasible to use a single model for multiple tasks. With SAM, the latest large-scale vision model, users can produce masks for specific areas of interest via interactive clicking, bounding box drawing, or verbal instructions. Considerable attention has been directed to its zero-shot and few-shot abilities across diverse fields using natural images.
Some initiatives have also focused on the zero-shot potential of SAM concerning medical imaging. Nevertheless, SAM struggles to generalize across multi-modal and multi-object medical datasets, resulting in inconsistent segmentation accuracy across datasets. This difficulty arises from a significant domain disparity between natural and medical images. This issue can be traced back to the data acquisition methods: since medical images are gathered with specific clinical aims, they are captured using designated protocols and scanners and represented as various modalities (electrons, lasers, X-rays, ultrasound, nuclear medicine, and magnetic resonance). Consequently, these images are markedly different from real-world images due to their reliance on varied physics-based attributes and energy sources.
As illustrated in Figure 1, natural and medical images exhibit considerable differences in pixel intensity, color, texture, and other distributed characteristics. Since SAM is trained solely on natural images, it lacks the specialized knowledge required for medical imaging, rendering it impractical for immediate application within the medical field. Supplying SAM with medical knowledge poses challenges because of the high costs of annotation and variable quality of annotations. Preparing medical data requires subject matter expertise, and the quality of such data can greatly differ between institutions and clinical trials. These difficulties contribute to a significant variance in the volume of medical and natural images.
The bar chart presented in Figure 1 contrasts the data volume of publicly accessible natural image datasets with medical image datasets. For example, Totalsegmentor, the largest public segmentation dataset within the medical arena, has a considerable gap compared to Open Image v6 and SA-1B. In this research, their aim is to transition SAM from natural images to medical images. This transition will establish benchmark models and evaluation frameworks for researchers in medical image analysis, encouraging exploration and enhancement. To accomplish this objective, researchers from Sichuan University and Shanghai AI Laboratory have introduced SAM-Med2D, the most thorough investigation into applying SAM to medical 2D images.
Be sure to check out the Paper and Github. All Credit for This Research Goes To the Researchers on This Project. Additionally, do not forget to join our 29k+ ML SubReddit, 40k+ Facebook Community, Discord Channel, and Email Newsletter, where we distribute the latest AI research updates, exciting AI projects, and more.
If you appreciate our efforts, you will enjoy our newsletter.
Aneesh Tickoo is a consulting intern at MarktechPost. He is currently pursuing his undergraduate degree in Data Science and Artificial Intelligence from the Indian Institute of Technology (IIT), Bhilai. Most of his time is occupied working on projects aimed at leveraging the power of machine learning. His research interests include image processing, and he is enthusiastic about creating solutions around it. He enjoys connecting with individuals and collaborating on engaging projects.
Be the first to comment